The backbone of AI success: data engineering and workflow design in medical AI projects
- Radoslaw Gasiorek
- Apr 14
- 2 min read
In the AI ecosystem, success stories often spotlight advanced algorithms or groundbreaking models. However, the unsung heroes are the data strategies and workflow architectures that enable AI systems to perform effectively in real-world scenarios. Drawing from Montrose Software's internal best practices and standards, this article explores how data quality and structured workflows amplify AI's impact, particularly in medical prediction models.
From data chaos to clarity: the journey begins
At the heart of any AI project lies the data. In medical AI, datasets are often riddled with inconsistencies: missing health metrics, varied formats, and unstructured text entries. Montrose's internal standards emphasize three critical principles for transforming messy data into a usable asset:
Data cleaning & imputation
Missing values, such as patients' weight or blood pressure, can cripple predictive accuracy. By employing imputation techniques like Multivariate Imputation by Chained Equations (MICE), Montrose ensures datasets are robust without introducing bias. For instance, this approach improved a diabetes risk model's reliability by filling gaps in health records.
Semantic standardization
Doctors' notes often describe the same condition in different terms—“high blood pressure” versus “hypertension.” Using AI-driven embeddings for semantic similarity detection, these variations are standardized, increasing diagnosis accuracy. A project focused on hypertension saw a 25% improvement in identification rates after applying these methods.
Normalization
Units for medical metrics like weight or height may vary across regions. A systematic conversion process ensures uniformity, reducing confusion and enhancing model performance.
Why workflow matters: the Montrose AI framework
Montrose's internal workflow emphasizes iterative cycles of testing, validation, and refinement. This structure supports continuous improvement and scalability. The process involves:
Baseline establishment: Initial model iterations act as a performance benchmark.
Iterative Model Training: Feedback loops allow fine-tuning using real-world data.
Validation and Deployment: Models are rigorously tested before integration into production systems.
This workflow ensures AI solutions are not only accurate but also practical, balancing precision and recall to minimize false positives in critical scenarios like disease diagnosis.
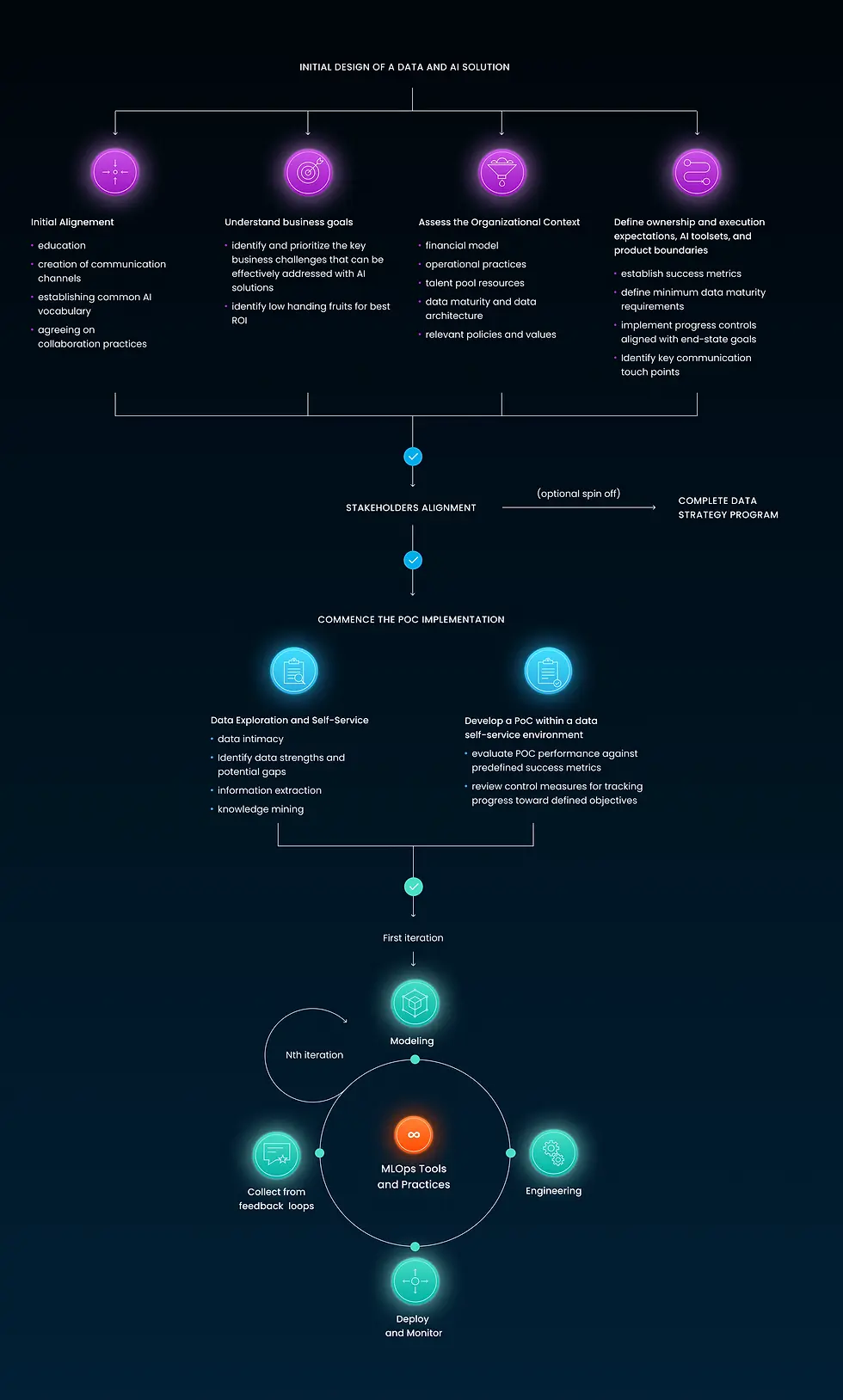
A case in point: medical data optimization
One recent project aimed to enhance diabetes risk prediction using data from anonymized data of 49,000 patients. Initial models struggled due to fragmented datasets and unstructured text inputs. By implementing Montrose's best practices:
Data engineering boosted model accuracy:
Standardized and normalized data inputs enhanced the Random Forest model's precision and recall without the need for overly complex tuning.
Cluster analysis improved personalization:
Patients were segmented into distinct clusters based on lifestyle and demographics, enabling tailored healthcare interventions.
The result? Predictive accuracy surged by 20%, and healthcare providers could proactively address risks within targeted patient groups.
Lessons learned: why data quality trumps model tuning
In AI, it's tempting to invest heavily in tweaking algorithms. Yet, as Montrose's projects demonstrate, robust data engineering often yields greater gains. Clean, consistent, and semantically unified datasets empower even basic models to outperform their sophisticated but poorly-fed counterparts.
Closing thoughts: invest in the foundation
The path from proof-of-concept to production in AI is paved with meticulous data preparation and thoughtful workflow design. Montrose's experience underscores that investing in these foundational elements is not a luxury—it’s a necessity.
Whether you're tackling medical optimization, disease classification, or broader AI challenges, remember: success starts with the data. And the journey to “done, done, done” relies on a well-structured roadmap, driven by industry-leading best practices.